Manuela 2.0: AI Revolutionizes Technical Documentation
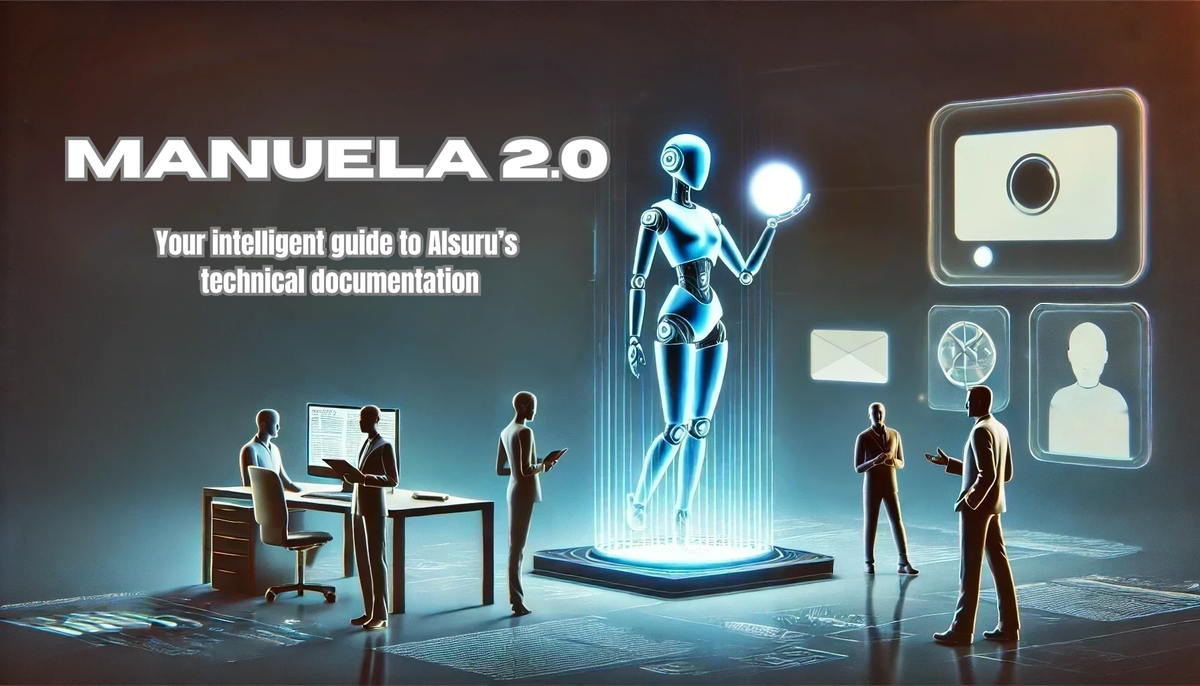
Imagine having to search for specific information in over 130 pages of technical documentation. How long would it take you? What if you could simply ask a question and get an immediate answer?
At Memori, we tackled this challenge by developing Manuela 2.0, a revolutionary system that transforms static documentation into an intelligent virtual assistant.
Thanks to its integration with AIsuru, Manuela 2.0 doesn't just search for information: it understands users' questions and provides precise, contextualized answers.
The Architecture That Makes the Difference
Manuela 2.0 isn't just a document search engine. It's a sophisticated system that combines four key components to ensure precise, contextualized answers:
- Gitbook documentation:
- A comprehensive documentation organized in structured thematic chapters and updated content. LINK.
- Intelligent scraping system:
- An automatic documentation processing system that cleans the data and divides it into files based on chapters;
- Web service to expose hapters:
- A web service that makes chapters available through a URL accessible by AIsuru functions.
- AIsuru platform:
- An AI system that manages the knowledge base, dynamically retrieves necessary content, and generates contextual responses for users.
Our Results Speak for Themselves
Preliminary tests of Manuela 2.0 have shown significant improvements over the previous version:
Improved accuracy
- 36.37% increase in completely correct responses;
- 24.24% reduction in partially correct responses;
- 12.12% decrease in incorrect responses.
Impact on User Experience
- Immediate responses instead of long manual searches;
- Greater precision in provided information;
- Natural and conversational interaction with documentation.
These numbers translate to an overall accuracy close to 80% of fully correct responses, an extraordinary result considering that initial tests have highlighted areas for further optimization of this outcome.
Beyond Testing: Refining the User Experience
During preliminary internal testing of Manuela 2.0, we identified and resolved several critical issues that are typical when implementing AI-queryable documentation.
During internal testing, the team identified three main areas requiring attention. Experience has taught us that these areas are often the most common challenges when transforming traditional documentation into an AI-queryable system.
User Experience
The first version of Manuela 2.0 tended to provide overly verbose and generic answers. This is a common problem: AI tries to be exhaustive but risks overloading users with information. We therefore worked to make responses more concise and direct, allowing Manuela 2.0 to ask users questions when their request wasn't sufficiently clear.
Response Contextualization
A documentation AI must understand exactly what the user is looking for, without simply repeating what the documentation says. We identified and corrected this behavior, implementing a greater focus on question objectives and eliminating superfluous information. Response contextualization became a priority to ensure users received information relevant to their specific case.
Technical Aspects
Manuela 2.0 struggled to locate some specific parts of the documentation, especially when content wasn't optimally structured. Additionally, the AI tended to give imprecise explanations when baseline information wasn't sufficiently clear or when concrete examples were missing. In some cases, the system failed to effectively connect related concepts that were present in different sections of the documentation. These challenges require careful optimization of both content structure and system architecture.
The lessons learned during this phase allowed us to prepare a more robust and effective system before releasing it for beta testing to a select audience.
The Path to Release
Releasing an AI system like Manuela 2.0 requires a structured approach: after internal testing that allowed us to achieve accuracy close to 80%, we began the beta testing phase with a selected group of internal users.
This phase is crucial for verifying how real users interact with the system and ensuring that responses maintain a high level of reliability before public release on the AIsuru platform.
Only after validating performance with this user group and implementing the final necessary refinements will we proceed with the system's public release. This gradual approach will allow us to further perfect the system based on real feedback and concrete use cases.
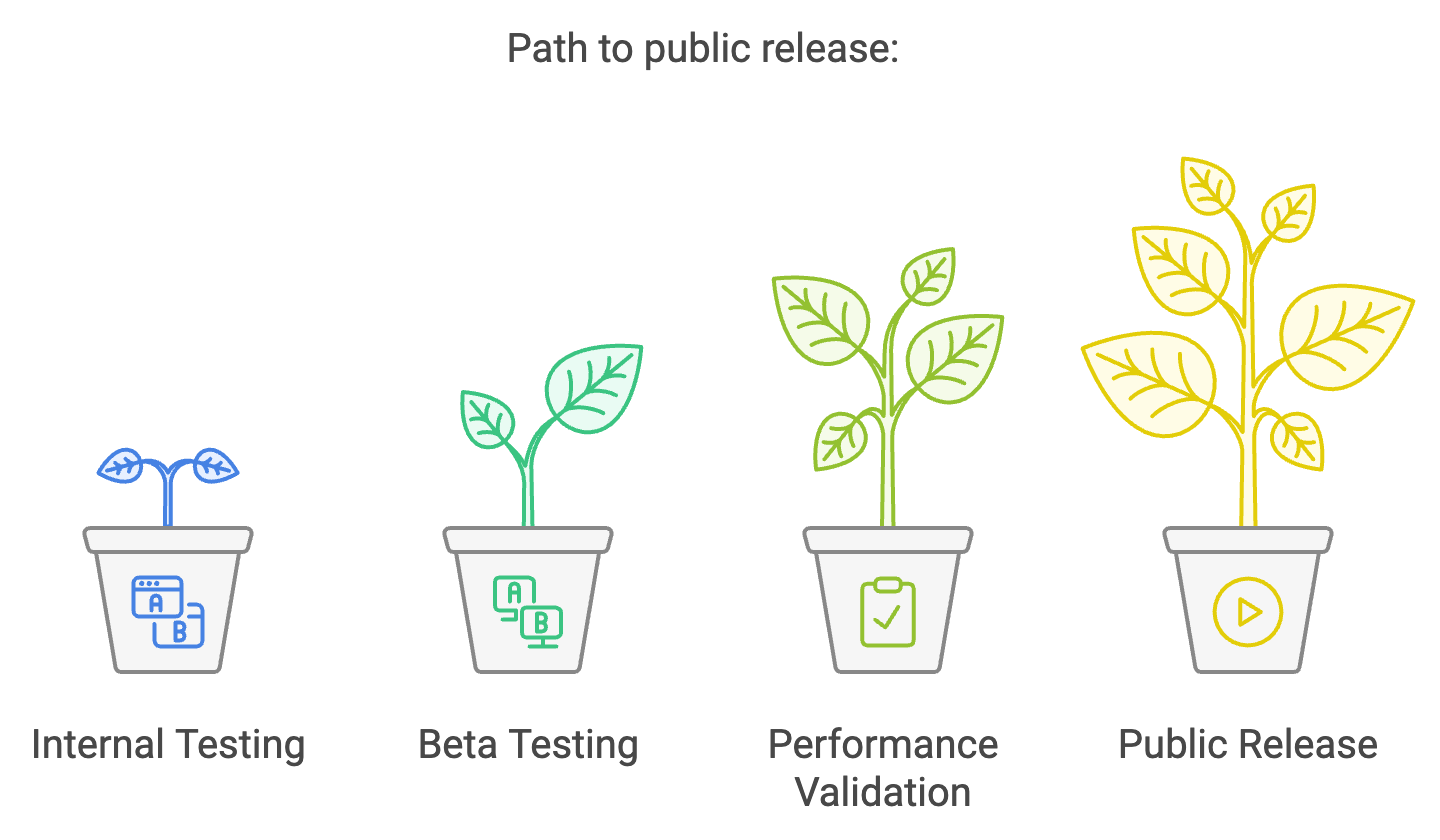
Transform Your Documentation with Manuela 2.0
Manuela 2.0 represents the future of technical documentation: a system that not only provides information but understands and meets user needs.
Do you have complex documentation that you'd like to make more accessible to your users?
Start Today
- Request a call with Memori to explore your documentation;
- Experience the power of intelligent documentation;
- Transform your users' experience forever. Click here to contact us: link.